Identification of oceanographic features that rule the biological productivity in the coastal zone of Argentina Patagonia – A study based on remote sensing data
August 8, 2018
PhD in Biology (2017), Dep. of Biology. Universidad Nacional del Comahue – Centro Regional Universitario Bariloche. Advisors: Dra. Ana I. Dogliotti and Dra. Gabriela N. Williams
Data from satellite images help solve a problem regarding the study of large systems: the difficulty to perform high frequency and big-scale surveys. These images became an alternative to the traditional and limited field surveys, despite having some restrictions. There is a wide variety of satellite systems in terms of both technical and practical characteristics. It is fundamental to choose wisely the type of data to use in order to answer different kinds of questions. This work uses diverse systems and methodologies aiming at responding three different questions and at different temporal and spatial scales.
A 9 year time series (2000-2008) of NOAA-AVHRR SST (sea surface temperature) images plus a 7 year time series (2000-2006) of OrbView2-SeaWiFS chlorophyll-a (Cla-sat) images (1.1 km) were used for the characterization of the San Jorge Gulf (GSJ), Argentina. The spatio-temporal patterns of SST and Cla-sat led to the identification of three frontal systems: North, South and External, which were then associated with the life cycle and fishery of the Argentinean red shrimp (Pleoticus muelleri), main target species of the industrial fleet over the GSJ.
Another study focused on a small area along the coast of Chubut province, north of San Jorge Gulf, particularly over the subareas called Bahía Camarones and Cabo Dos Bahías, which have a wide subtidal region with great abundance of the brown algae Macrocystis pyrifera or kelp. A low cost and high efficiency technique was developed in order to monitor the spatio-temporal distribution and dynamics of these forests, with potential to be applied in any other geographical region. A series of 134 Landsat TM and ETM+ images with 30 m of spatial resolution belonging to the period 1975-2011 were used. Individual forest units were identified in each subarea through the normalized difference vegetation index (NDVI), and geographic information systems (GIS) tools were used to calculate the maximum area of such units, which were later corrected due to tidal effects.
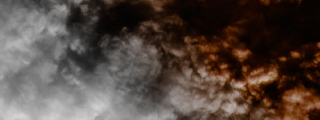